i help good people
build good brands.
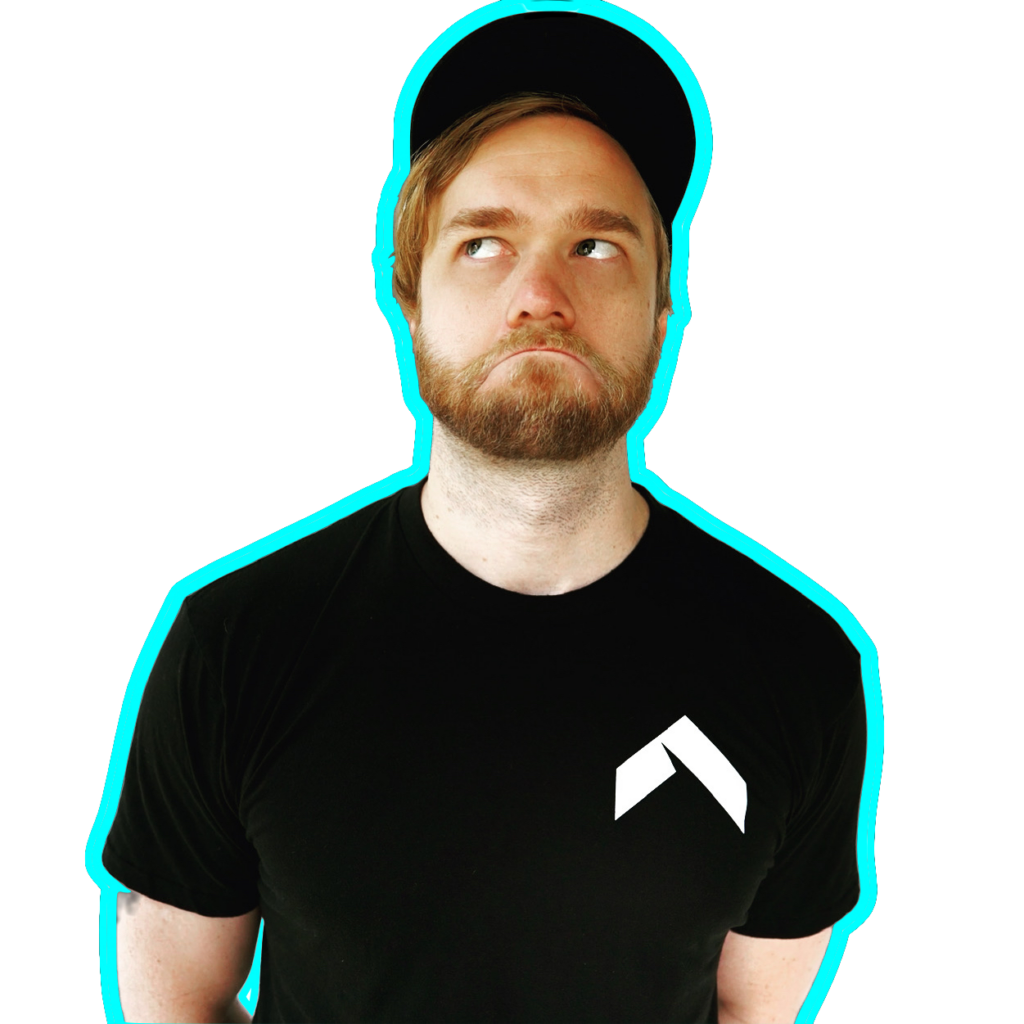
How to write content that ranks
Content Writing Mastery is a step-by-step course that shows you exactly how to write SEO optimized website content that
I’ve used to generate millions of visitors from search engines. All you have to do is follow along!
๐ Click here to check it out!
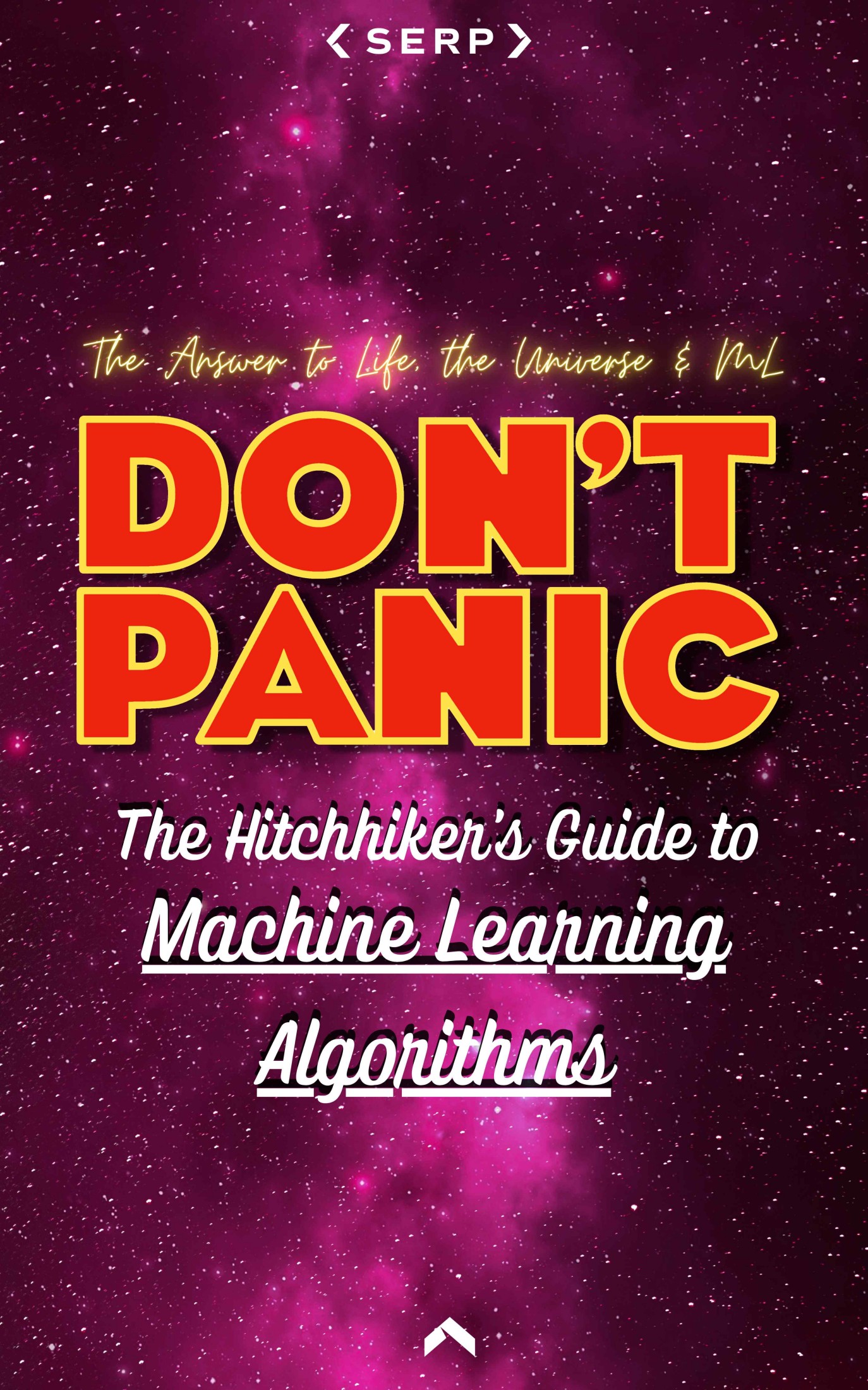
The Hitchhiker’s Guide to Machine Learning Algorithms:
100+ Machine Learning Algorithms Explained So Simply Even a Human Can Understand
Hello humans & welcome to the world of machines!
Specifically, machine learning & algorithms. We are about to embark on an exciting adventure through the vast and varied landscape of algorithms that power the cutting-edge field of artificial intelligence.
Machine learning is changing the world as we know it. From predicting stock market trends and diagnosing diseases to powering the virtual assistants in our smartphones and enabling self-driving cars, and picking up the slack on your online dating conversations. What makes this book unique is its structure and depth.
With 100 chapters, each dedicated to a different machine learning concept, this book is designed to be your ultimate guide to the world of machine learning algorithms. Whether you are a student, a data science professional, or someone curious about machine learning, this book aims to provide a comprehensive overview that is both accessible and in-depth.
Whether you are looking to expand your knowledge, seeking inspiration, or in pursuit of technical mastery, this book should sit on your coffee table and make you look intelligent in front of all invited (and uninvited) guests.